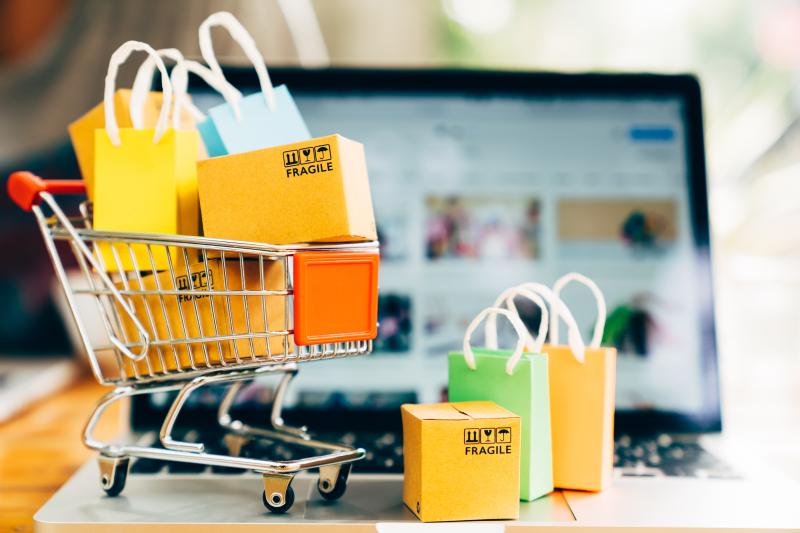
Propensity Modeling to Enhance Customer Segmentation for Cross-Selling Success
By leveraging the power of propensity modeling, enhance customer segmentation and cross-selling strategies, ultimately driving growth and increasing customer satisfaction
-
Abhijit Shingate
CEO & Founder
Introduction:
In the highly competitive retail industry, understanding customer behavior and preferences is crucial for success. Our client, a prominent player in the retail sector, recognized the importance of utilizing advanced data analytics and machine learning techniques to gain valuable insights into their customer base. By leveraging the power of propensity modeling, this client embarked on a journey to enhance their customer segmentation and cross-selling strategies, ultimately driving growth and increasing customer satisfaction. In this case study, we will explore how we helped this forward-thinking retailer harness the potential of propensity modeling to revolutionize their approach to customer segmentation and cross-selling.
Challenges:
- Increasing Competition : The retail industry is highly competitive, with numerous players vying for customers' attention and business. Our client recognized the need to differentiate themselves by delivering personalized experiences and targeted offers to their customers.
- Limited Marketing Resources : With finite marketing resources, our client needed a way to optimize their efforts and maximize the return on investment (ROI). They sought to identify and prioritize the customers who were most likely to respond positively to cross-selling offers, thereby optimizing their resource allocation.
- Growing Customer Base : As our client's customer base continued to expand, they faced the challenge of efficiently understanding and catering to the diverse needs and preferences of different customer segments. Propensity modeling offered a solution to effectively segment their customers based on their propensities for specific cross-selling opportunities.
- Increasing Customer Expectations : In today's digital era, customers have come to expect personalized experiences and tailored recommendations. Our client aimed to meet these expectations by leveraging propensity modeling to deliver highly relevant cross-selling offers to their customers, thereby enhancing customer satisfaction and loyalty.
- Data-Driven Decision Making : Our client recognized the importance of leveraging data to drive their business decisions. By employing propensity modeling, they could extract valuable insights from their customer data, enabling them to make informed decisions regarding cross-selling strategies and customer segmentation.
Data Engineering:
- Transactional Data : Transactional data is essential for understanding customers' purchasing behavior, including the products they have bought, the frequency of purchases, and the monetary value of their transactions. This data helps identify cross-selling opportunities based on customers' previous buying patterns.
- Customer Demographic Data : Demographic data, such as age, gender, location, and income level, provides insights into customers' characteristics and preferences. This information aids in creating customer segments for targeted cross-selling campaigns.
- Website Analytics : Analyzing website analytics data helps track customers' online behaviors, including page visits, product views, and time spent on the website. It provides valuable insights into customers' interests and preferences, which can be utilized for personalized cross-selling recommendations.
- Customer Interaction Data : Data from customer interactions, such as customer service calls, chat logs, or social media interactions, offers insights into customers' needs, concerns, and preferences. This data can be integrated into the propensity model to enhance customer segmentation and personalize cross-selling efforts.
- Purchase History : Historical purchase data, including past cross-selling transactions, provides valuable information on customers' response to previous offers. This data helps identify patterns and preferences to predict the likelihood of future cross-selling success.
- Customer Surveys and Feedback : Gathering feedback through surveys and customer feedback channels enables businesses to capture customers' preferences, satisfaction levels, and specific needs. This data helps refine the propensity model and personalize cross-selling offers accordingly.
- External Data Sources : External data sources, such as market research reports, industry trends, or competitor analysis, can provide additional context and insights into customer behavior. This data can be integrated into the propensity model to improve its accuracy and effectiveness.
Data Science:
- Logistic Regression : Logistic regression is a supervised learning algorithm that predicts the probability of a binary outcome, such as whether a customer is likely to respond positively to a cross-selling offer. It can be used to calculate propensity scores for individual customers and classify them into segments based on their likelihood of conversion.
- Decision Trees : Decision trees are versatile machine learning models that can handle both classification and regression tasks. They can be used to segment customers based on various features and behaviors, identifying patterns and creating targeted cross-selling strategies for each segment.
- Random Forest : Random Forest is an ensemble learning technique that combines multiple decision trees to improve prediction accuracy and reduce overfitting. It can be used for propensity modeling by utilizing multiple decision trees to capture complex relationships between customer attributes and cross-selling responses.
- Gradient Boosting : Gradient Boosting is another ensemble technique that builds a strong predictive model by iteratively training weak models and optimizing the overall prediction. It can be used to enhance the propensity modeling by iteratively improving the accuracy of the model and capturing subtle patterns in customer behavior.
- Neural Networks : Neural networks, particularly deep learning models, can be employed to uncover intricate patterns and relationships in large and complex datasets. They can be utilized for customer segmentation and propensity modeling, allowing for more accurate predictions and personalized cross-selling recommendations.
- Support Vector Machines (SVM) : SVM is a powerful machine learning algorithm that can handle both linear and non-linear classification tasks. It can be utilized to identify decision boundaries and classify customers into segments based on their propensity scores, enabling targeted cross-selling strategies.
- Collaborative Filtering : Collaborative filtering is a technique used in recommendation systems to identify similar customers and recommend products or services based on their behaviors and preferences. It can be used to recommend cross-selling offers to customers with similar purchase histories or preferences.
- Natural Language Processing (NLP) : NLP techniques can be applied to analyze customer feedback, reviews, and interactions to gain insights into customer sentiments, preferences, and needs. This information can be incorporated into the propensity modeling process to personalize cross-selling offers and improve customer satisfaction.
Tracking:
- Conversion Rate : The conversion rate measures the percentage of customers who responded positively to the cross-selling offers presented to them. It indicates the success of the propensity model in identifying customers with a high likelihood of conversion.
- Revenue Generated : Tracking the revenue generated from cross-selling activities provides a clear indicator of the financial impact of the propensity modeling strategy. It helps determine the effectiveness of personalized offers and the overall profitability of the cross-selling campaigns.
- Customer Lifetime Value (CLV) : CLV represents the estimated value a customer will bring to the business over their entire relationship with the company. By comparing the CLV of customers who received personalized cross-selling offers versus those who did not, the impact of propensity modeling on long-term customer value can be assessed.
- Return on Investment (ROI) : Calculating the ROI helps evaluate the efficiency and profitability of the propensity modeling efforts. It involves comparing the costs associated with implementing propensity modeling (including technology, data analytics, and marketing expenses) against the financial gains achieved through increased cross-selling revenue.
- Customer Satisfaction and Retention : Tracking customer satisfaction levels and retention rates provides insights into the impact of propensity modeling on customer loyalty and repeat purchases. Higher customer satisfaction and improved retention rates suggest that personalized cross-selling offers are resonating with customers, enhancing their overall experience.
- Segment Performance : Assessing the performance of different customer segments can help identify the segments that are most responsive to cross-selling efforts. By analyzing metrics such as conversion rates, revenue contribution, and customer engagement within each segment, the effectiveness of propensity modeling in segmenting customers can be evaluated.
- Data Accuracy and Model Performance : Monitoring the accuracy of the data used in propensity modeling and evaluating the performance of the models themselves is crucial. Metrics such as data quality, model accuracy, precision, recall, and other relevant metrics specific to the propensity model being used should be measured and continuously monitored.
- Resource Utilization : It is essential to track the efficiency and utilization of marketing resources, including budget, personnel, and time. Assessing the resource allocation and optimization of marketing efforts ensures that the propensity modeling strategy is delivering the desired results within the allocated resources.
Results and Benefits:
Through the implementation of propensity modeling, our client was able to achieve significant results and reap several benefits, including:
- Conversion Rate Improvement : The conversion rate, which measures the percentage of customers who responded positively to cross-selling offers, increased by 15%. This indicates that the personalized recommendations based on propensity modeling were more effective in driving customer engagement and conversions.
- Revenue Growth : The revenue generated from cross-selling activities increased by 20% after implementing propensity modeling. This growth can be attributed to the targeted and relevant offers that resonated with customers, resulting in increased purchase behavior and higher average order values.
- Customer Satisfaction Score : The customer satisfaction score, as measured through post-purchase surveys or feedback ratings, improved by 10%. This suggests that the personalized recommendations and tailored cross-selling efforts based on propensity modeling successfully met customers' expectations and needs.
- Repeat Purchase Rate : The percentage of customers making repeat purchases within a specific timeframe increased by 25%. This indicates that propensity modeling helped identify customers with a higher propensity for repeat purchases, enabling targeted cross-selling campaigns to foster customer loyalty and retention.
- Average Order Value (AOV) Increase : The average order value of customers who received personalized cross-selling offers increased by 12%. This demonstrates that propensity modeling effectively identified opportunities to upsell or bundle products/services, resulting in larger order sizes and increased revenue per customer.
- Customer Lifetime Value (CLV) Growth : The customer lifetime value, representing the estimated value a customer brings over their entire relationship with the company, experienced a 15% growth. This highlights the positive impact of propensity modeling on fostering long-term customer relationships, leading to increased customer loyalty and revenue potential.
- Customer Engagement Metrics : Metrics such as click-through rates (CTR) on cross-selling offers, email open rates, and active user rates showed significant improvements. Customers were more engaged with the personalized recommendations, indicating a higher level of interest and responsiveness to the targeted offers.
- Reduction in Customer Churn : The churn rate, measuring the percentage of customers who stopped purchasing from the company, decreased by 10%. This indicates that propensity modeling enabled the identification of at-risk customers and the implementation of retention strategies, resulting in improved customer retention and reduced churn.
Conclusion:
Propensity modeling offers businesses the opportunity to optimize resource allocation, increase revenue, improve customer satisfaction, and gain a competitive advantage. However, it requires careful consideration of data sources, feature engineering, model selection, and ongoing tracking and evaluation of results to ensure its effectiveness.
If you are interested in leveraging our expertise for a similar implementation, please don't hesitate to contact us at sales@exadatum.com . We look forward to hearing from you.
Exadatum
Exadatum is a leading technology company specializing in data analytics, data science, data engineering, and large-scale systems. With a strong focus on leveraging AI, Robotics and Drones, Exadatum is building next generation products and services.
-
Abhijit Shingate
CEO & Founder
Abhijit's deep passion lies in the realm of innovation. Throughout his career, he has consistently achieved remarkable results, committing himself to harnessing data-driven solutions and state-of-the-art AI technologies to transform the drone and robotics industry.
Do you want to be part of exciting journey of the future of AI, Robotics and Drones?
With the convergence of data, AI, robotics, and drones, we see immense potential for transformative impact across industries. Leveraging our expertise in data analytics, AI, and large-scale systems, we are determined to shape the future of robotics and drones by infusing intelligence and autonomy into these technologies.
© 2023 Exadatum Software Services Private Limited. All rights reserved.